Machines can be programmed to mimicking human intelligence and learn by examining patterns in data results. It is a result of collective predictive analysis, continuous analytics and prescriptive analytics. al-time, post or pre-event data analysis processing can increase the accuracy of predictions and act accordingly with respect to the commands input by the external stimulus. Stimulating this artificial intelligence is a result of collective predictive analysis, continuous analytics and prescriptive analytics. Most of the current applications, or devices are completely dependent on the AI, as it gives a better interactive environment which is impelling.
Just the mention of AI and the brain invokes pictures of Terminator machines destroying the world. Harnessing the power of new technologies for good is one of our key challenges and the potential of these advances as well as their application to the world’s most pressing problems is immense. Artificial Intelligence is the simulation of human intelligence done by machines programmed by us. The machines need to learn how to reason and do some self-correction as needed along the way. Now that we have detailed algorithms which Artificial Intelligence systems can make use of, they can perform huge tasks faster and more efficiently. Machine Learning and Deep Learning are just ways to achieve Artificial Intelligence.
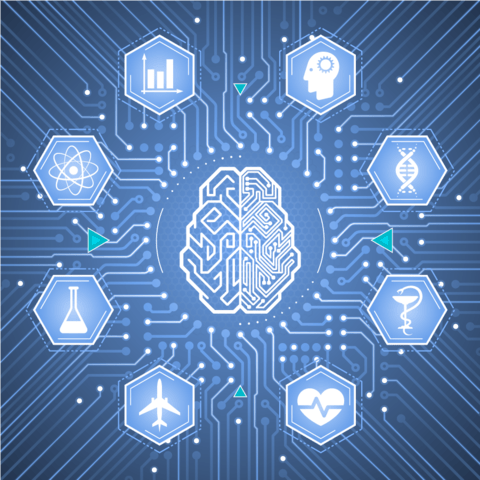
As with most changes in life, there will be positive and negative impacts on society as artificial intelligence continues to transform the world we live in. How that will balance out is anyone's guess and up for much debate and for many people to contemplate. As an optimist at heart, I believe the changes will mostly be good but could be challenging for some. Here are some of the challenges that might be faced (and we should be thinking about how to address them now) as well as several of the positive impacts artificial intelligence will have on society.
Ethics should be a central consideration for companies and individuals developing AI, in order to create positive meaningful change for society. Those who have considered the ethical implications of their products and services, and who monitor, manage and communicate effectively about them, will have a competitive edge.
The true value of data lies in connecting many pieces together from various sources to create a holistic view. That's where AI becomes critical. It works somewhat like a research assistant. You tell it that you have a theory about what might be learned by cross-referencing different kinds of data and set the AI loose to crawl all that data and learn on its own, looking for complex patterns that would escape even brilliant humans. And unlike a human, an AI bot can absorb and learn from massive floods of data in an instant.
The use of Big Data has continued to grow and mature, with some organizations reaping considerable rewards. The processing of Big Data has recently advanced to a new level of evolution, in the form of AI (Artificial Intelligence) platforms. AI platforms promise significant impact (and disruptions) over the next decade. The use of AI to process massive datasets will bring previously unknown improvements to Business Intelligence and Analytics among innumerable other technologies.
AI, Machine Learning, Deep Learning, General AI
AI is constructed on a set of algorithms that try to imitate human intelligence. Machine learning is one of these algorithms and deep learning is a sub-skill of a machine learning technique. Machine learning consumes data and uses statistics to better learn the data, in turn improving the ability to solve the task. Machine learning is made up of both supervised and unsupervised learning. Supervised learning learns on a labeled dataset, allowing you to produce outputs based on previous data. In contrast, Unsupervised learning uses unlabeled data to learn on and discover unknown patterns in data.
Deep learning, also known as deep neural learning, is a type of machine learning technique that tries to imitate the human brain by inputting data through a biological inspired neural network that contains a number of hidden layers. Through the hidden layers, the data is processed making connections and creating patterns. General AI has a bit more complexity to it and tries to mirror human intellect using its ability to learn and apply the knowledge learnt to solve problems.
Examples of AI application:
- Speech recognition: This is the process of enabling a computer to recognise spoken words and have the ability to respond, for example; Siri.
- Natural Language Processing: This enables software to understand, interpret and generate the human language, for example; language translation and email filtering.
- Image recognition: This process can classify text, people, and objects as well as moving images. Examples of this are; fingerprint ID systems, self-driving cars, and face recognition.
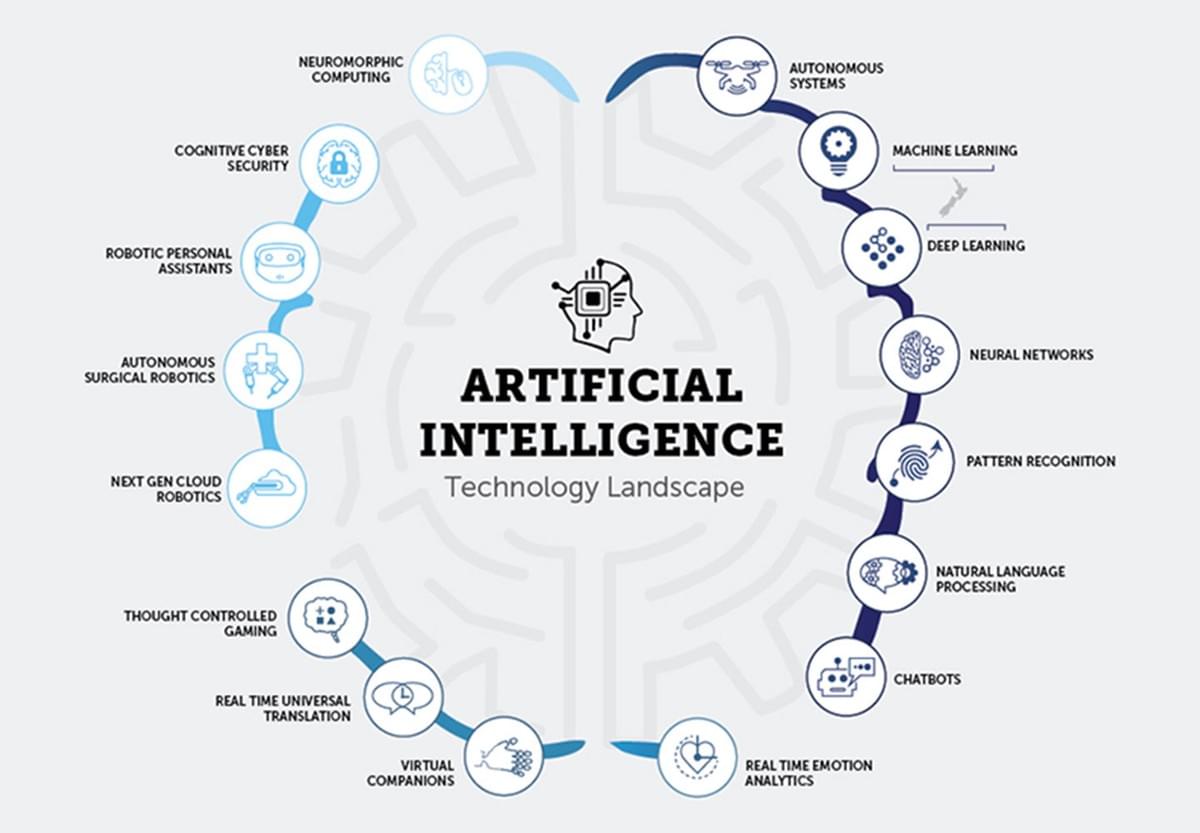
Cognitive systems have the ability to analyze, interpret, reason and learn, thus augmenting human intelligence, and they are able to do so without constant human involvement. With the ability to understand the context behind the content, cognitive computing is taking Big Data analytics from data-driven to value-driven. As we unleash more possibilities and opportunities for data through technological advances, we are able to not only turn data into information, but also to transform it into knowledge and even wisdom.
Relying on the foundation of storage and data at scale, here are four key areas where big changes are happening that will propel cognitive computing forward:
Less Human Interaction
As cognitive computing becomes more mainstream and more mature through deep learning, neural nets, pattern recognition and natural language, processing cobots (Collaborative Robots) are now performing more tasks than ever. You can see this in manufacturing plants and virtual assistants in human-less grocery stores, automated eateries, and, of course, driverless car services (semi-autonomous driving).
Data-Driven Programming
Modern-day chatbots deliver simulated conversation by leveraging machine learning and picking up on conversational tendencies to mimic human interaction when delivering a service. Chatbots are also growing as a robust tool to gather business intelligence and advance artificial intelligence through responsive technology. Many tech platforms are already helping developers build chatbots on their platform and businesses are now increasingly using chatbots through popular messengers as front ends for cognitive tools. As this evolves we may see chatbots becoming the new websites and messaging apps the new browser.
Edge Computing
With some analysts estimating IoT devices may reach 50 billion devices by 2020, the bandwidth available to support connected devices will not be sufficient to keep up. Many IoT devices require high-speed data processing and analytics with short response times (think of the use cases of vehicle-to-vehicle communication or medicine where milliseconds matter) that are difficult to meet by sending data to a centralized cloud. Edge and fog computing solve this pain point by placing processes and resources at the edge of the cloud, while data is actually stored within the cloud. This solves the bandwidth problem and results in faster local processing, delivering near real-time analytics.
Serverless Computing
Function-as-a-Service (FaaS) and serverless architectures are shifting how companies think about infrastructure that is lightweight and can be triggered on an action. Developers can now focus on an application without needing to build it for a specific configuration, scaling it up or down, or spinning up a VM or containers – it all happens through automatic sequences. IoT edge devices and gateways will gain great value from these architectures, here, too, enabled by less human intervention.
More Data and More Value Ahead
Artificial Intelligence (AI), machine learning, and deep learning are not new, but now we are able to harness the data which is required to make these algorithms more intelligent through large data lakes and data oceans, enabled by high-density storage. Algorithms are becoming a commodity and the data that trains them is a strategic asset. Computing power enabled by GPUs is able to handle deep algorithms and help with unsupervised learning, the open sourcing of deep learning frameworks, mobile computing, attention marketing and, most importantly, the rise of Augmented Intelligence.
The power of data is undeniable, and companies are finding ways to generate more value from it, enabled by the combination of people and machines. Underlying storage, network, and compute infrastructure is what powers the possibilities of data; choosing the right building blocks is what will open up new opportunities for businesses, services, and innovation. With the onslaught of data, density, simplicity and affordability are key. Employing cost-effective, scalable and powerful solutions such as high-density Big Data flash platforms like InfiniFlash™ for processing and analytics, or highly scalable modular storage systems for cloud storage, enables businesses to address the growing demands of data and enables new possibilities of delivering value.